OSFI-FCAC Risk Report - AI Uses and Risks at Federally Regulated Financial Institutions
Table of contents
1 Introduction
1.1 Background and purpose of report
As advances in artificial intelligence (AI) continue with unprecedented speed, the Office of the Superintendent of Financial Institutions (OSFI) and the Financial Consumer Agency of Canada (FCAC) are monitoring the evolving risk landscape and advocating for responsible AI adoption. In 2022, OSFI co-hosted the Financial Industry Forum on Artificial Intelligence (FIFAI) with the Global Risk Institute (GRI). The public report "A Canadian perspective on responsible AI" outlines the EDGE principles (Explainability, Data, Governance, Ethics) as a cornerstone for responsible AI use.
In December 2023, OSFI and FCAC shared a voluntary questionnaire to federally regulated financial institutions (financial institutions) requesting feedback on AI and quantum computing preparedness. In April 2024, OSFI issued a Technology Risk Bulletin on Quantum Readiness to financial institutions.
Following from that bulletin, this report outlines key risks that arise for financial institutions from AI, supported by findings from the questionnaire and insights from external publications. These risks can come from internal AI adoption or from the use of AI by external actors.
It also presents certain practices that can help mitigate some of the risks. These are not meant to serve as guidance but can be positive steps in a financial institution's journey to manage the risks related to AI.
Please note that we use "AI" to refer to a broad range of different machine learning algorithms, model methodologies, and systems. When reading this document, keep in mind that possible risks and consumer issues may differ greatly, depending on the specific type of AI models and algorithms used.
1.2 Use of AI at financial institutions
The data shown in the following infographic are from the AI/Quantum questionnaire.
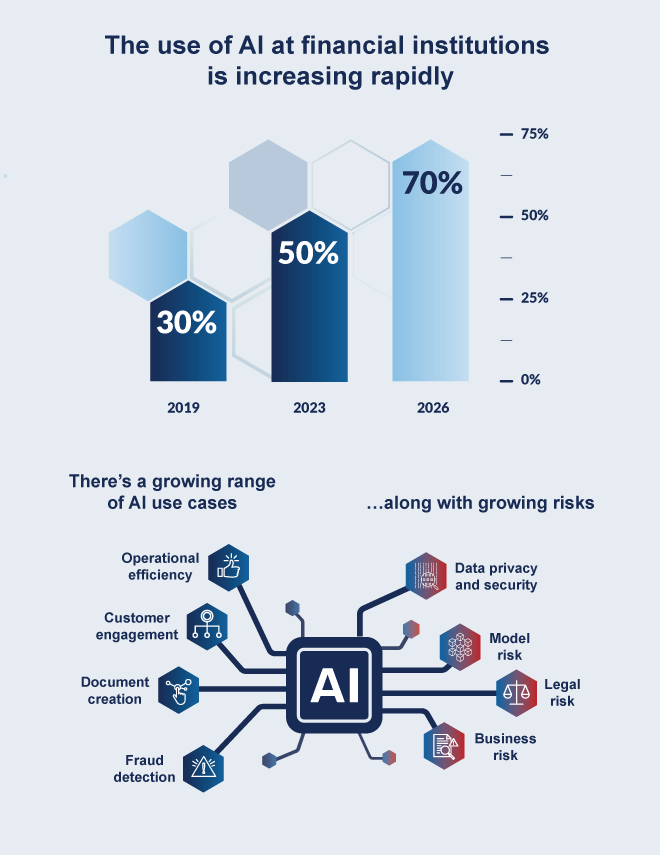
Use of AI at financial institutions - Text version
The use of AI at financial institutions is increasing rapidly:
- In 2019, approximately 30% of financial institutions
- In 2023, approximately 50% of financial institutions
- By 2026, 70% of financial institutions expected to be using AI
There's a growing range of AI use cases along with growing risks.
Top AI use cases:
- operational efficiency
- customer engagement
- document creation
- fraud detection
Top risk of AI use:
- data privacy and security
- model risk
- legal risk
- business risk
1.3 Executive summary
AI is affecting the way financial institutions conduct their business, run their operations, and manage risks. The potential benefits include enhancing customer engagement and risk management, improving decision making, and driving operational efficiency. However, AI can also amplify traditional risks and expose new vulnerabilities. It is becoming evident that the broader impacts of AI will affect the financial system whether financial institutions choose to adopt the technology or not.
Internal risks from AI
The questionnaire shows a significant increase in investments in AI and in the use of AI models since we last surveyed the industry in 2019. The range of use cases, number of staff working on AI, and approaches to managing risks have all increased. Generative AI (gen AI)Footnote 1, with its flexibility and ease of use, has played a key role in this.
Financial institutions are now using AI for more critical use cases, such as pricing, underwriting, claims management, trading, investment decisions, and credit adjudication. The use of AI may amplify risks around data governance, modeling, operations, and cybersecurity. Third-party risks increase as external vendors are relied upon to provide AI solutions. There are also new legal and reputational risks from the consumer impacts of using this technology that may affect financial institutions without appropriate safeguards and accountability.
Financial institutions are also facing competitive pressures to adopt AI, leading to further potential business or strategic risks. They need to be vigilant and maintain adaptable risk and control frameworks to address internal risks from AI.
External risks from AI
AI systems continue to advance with startling speed. Gen AI models are already performing many tasks at human levels.Footnote 2 Malicious actors are leveraging gen AI to execute new and sophisticated attack strategies against financial institutions. As gen AI can facilitate the design and the speed of such attacks, it may change the economic incentives for threat actors who have traditionally focused more on larger institutions. The lower cost of attack execution may make smaller institutions more attractive targets.
Similarly, fraud instances are increasing and becoming harder to identify.Footnote 3 The widespread reliance on AI could accelerate market and liquidity risks, causing increased volatility and feedback loops in the financial markets.
AI adoption can also amplify geopolitical risks associated with misinformation and disinformation. Furthermore, the medium-term macroeconomic implications of AI adoption, affecting labour markets and industries, could increase credit risk.
All financial institutions, even those not implementing AI models, need to be aware of how the risk environment is changing. Given the rapid increase in AI risks and diverse approaches to managing them, financial institutions need to actively manage gaps and deficiencies.
OSFI's and FCAC's views on the risks
OSFI and FCAC recognize that addressing AI risks can be challenging. There may be difficult trade-offs, such as balancing data privacy and governance concerns with the need for models to be explainable, transparent, and unbiased. We are working together to actively monitoring the evolving risk environment and supporting best practices at financial institutions as they navigate the wide range of AI-related risks. We regularly collaborate and share information with peer regulators globally, as well as with other governmental agencies and departments. We acknowledge several broad AI guidelines or codes of conduct, issued by the Government of Canada, that apply to the use of AI in finance (the Voluntary code of conduct on the responsible development and management of advanced generative AI Systems; the Principles for responsible, trustworthy, and privacy-protective generative AI technologies; the Directive on Automated Decision-Making; and the Artificial Intelligence and Data Act).
OSFI and FCAC's respective regulatory frameworks already include guidance covering many prudential areas of concern affected by AI applications. This includes, for example, consumer protection laws and regulations, guidance on model risk management,Footnote 4 third-party risk management,Footnote 5 cybersecurity,Footnote 6 and operational resilience,Footnote 7 all of which are technology-neutral.
2 Internal AI activity
AI usage will continue to increase and affect all areas of financial institutions. Many questionnaire respondents indicated that AI is becoming essential to their efforts to drive competitive advantage and enhance value creation.
2.1 Increase of AI adoption and investment
Seventy-five percent of the financial institutions that responded to the questionnaire plan to invest in AI over the next three years as it becomes a fundamental strategic priority. Many of them rely on third-party providers. Additionally, 70% plan to use AI models within this timeframe. Among those not planning to use AI models, some are nevertheless planning to assess AI-related enterprise risks in anticipation of potential future use cases. Globally, according to Fortune Business Insights, the total AI market is projected to grow from 621 billion USD in 2024 to 2.7 trillion USD by 2032.
2.2 Activities affected by AI models
AI is increasingly being used for core functions such as underwriting and claims management. Approximately a quarter of insurers identify underwriting or claims management as top AI use cases. Deposit-taking institutions (DTIs) are also expanding their use of AI for core functions, including algorithmic trading, liquidity management, credit risk activities, and compliance monitoring.
Many financial institutions use AI to identify fraud. This is particularly true for DTIs, many of which cite fraud detection as one of their top AI applications. Insurers are also using AI for this purpose. Cybersecurity is another common use case, often involving third-party providers. AI can assist with tasks such as detecting network anomalies, classifying emails for suspected phishing attempts or malware, and detecting potential instances of fraud.
Operational efficiency and customer engagement are two areas where AI use cases are having a significant impact, with more activities planned over the next three years. Customer engagement use cases include customer support chatbots, customer verification, marketing applications, and personalized financial advice.
Gen AI is a significant driver of the growing range of AI applications. This technology enables use cases such as coding assistance, text generation, document summarization, knowledge retrieval, and meeting transcriptions. Questionnaire responses identified several third-party gen AI solutions used, including ChatGPT, Microsoft Copilot, Azure OpenAI, GitHub Copilot, Amazon Web Services, and Llama models. Most financial institutions, particularly smaller ones, are at the prototype stage of gen AI adoption. Some are reviewing large language models (LLMs) for various business use cases, including customization for internal use and customer engagement applications. A few are still assessing the risks and benefits of gen AI.
3 Internal risks from AI
Because AI adoption affects various types of risk, it can be considered a transverse risk. It may amplify existing risks and introduce new ones as usage increases and the scale of data collection and models expands into new areas. Gaps in risk management can arise as skills, controls and procedures strive to keep up and adapt.
3.1 Data governance risks
Data-related risksFootnote 8 are viewed by respondents as a top concern about AI usage. These risks are present throughout the data lifecycleFootnote 9 and encompass data privacy, data governance, and data quality. Factors such as differing jurisdictional regulatory requirements, fragmented data ownership, and third-party arrangements can be challenging. Addressing AI data governance is crucial, whether through general data governance frameworks, specific AI data governance, or model risk management frameworks.
The improper use of synthetic data could significantly impact financial institutions. While some of them currently use synthetic data for modeling, others are considering it for future applications. Although adoption is still nascent, financial institutions need to develop standards to evaluate and ensure the fidelity of synthetic data used in AI systems.
3.2 Model risk and explainability
The risks associated with AI models are elevated due to their complexity and opacity. Unlike traditional model risks,Footnote 10 AI models typically have a much higher number of parameters, which are learned autonomously from data. In addition to infrastructure and data challenges, causal relationships between inputs and outputs are often indeterminable. As a result, the stability and the intricacy of AI models pose a significant challenge. Financial institutions must ensure that all stakeholders—including users, developers, and control functions—are involved in the design and implementation of AI models. A lack of communication between model developers and users, for instance, could lead to inappropriate specifications, flawed hypotheses or assumptions, and improper usage.
AI models must be explainable, to provide stakeholders with meaningful information about how these models make decisions. Financial institutions need to ensure an appropriate level of explanation to inform internal users/customers, as well as for compliance and governance purposes.
Explainability is even more challenging for gen AI than for traditional AI and machine-learning models. The rapid adoption of gen AI, including LLMs, increases the risks related to the explainability of these models. This is a fundamental challenge for deep learning models.
3.3 Legal, ethical, and reputational risks
Financial institutions would be well served by taking a comprehensive approach to managing the risks associated with AI. Narrow adherence to jurisdictional legal requirements could expose financial institutions to reputational risks. As the pace of innovation accelerates, navigating an uncertain regulatory environment can be challenging.
Consumer privacy and consent should be prioritized. Financial institutions must educate customers and clearly disclose when AI impacts them, and they must obtain proper consent to use customers' data. This includes being transparent about targeted sales practices.
Bias is a complex and nuanced topic that can be difficult to address in practice. Bias can persist even when personally identifiable information (PII) is removed. Sometimes what is perceived as bias is an unavoidable outcome of risk stratification on permissible variables. Discriminatory bias typically emerges when seemingly innocuous proxy variables (for example, name or postal code) are used to inadvertently infer protected attributes (for example, race or religion) or when models are trained on biased human decisions. This may negatively impact financial institutions in the form of reputational, legal, or business risks. Financial institutions need to proactively assess bias by, for example, continuously monitoring AI models that impact customers, especially regarding pricing or credit decisions. Ensuring data representativeness and involving multidisciplinary teams with diverse members can also help prevent bias.
Testing for bias presents a fundamental data dilemma, because doing so accurately would require collecting sensitive data on protected customer attributes such as race or religion. Even if customers agree to provide this data, it would increase data privacy risks. The European Union's new AI Act notably carves out an exception to the General Data Protection Regulation to collect PII data on characteristics such as race and gender for the purpose of de-biasing AI algorithms.
Failing to assume responsibility for bias and fairness with third-party models can increase legal and reputational risks. The lack of accountability for business activities, business functions, and services provided by third parties can have severe consequences if a model fails. Financial institutions should assess and mitigate these risks whenever customers may be impacted.
3.4 Third-party risks
Most financial institutions rely on third-party providers for AI models and systems. The dependency on large tech firms could present a concentration risk, as was illustrated during the July 2024 global IT outage with an estimated financial loss of $5.4 Billion. Even if concentration risks can be mitigated, operational disruptions and risks to data integrity still need to be monitored.
It is challenging to ensure that third parties comply with a financial institution's internal standards. This is due in part to the technical expertise required to assess this compliance, and due to third parties' desire to protect their intellectual property rights. However, all third-party activities and services should be performed in a safe manner, in compliance with applicable legislative and regulatory requirements, and in accordance with each financial institution's own internal policies, standards, and processes. Financial institutions are responsible for the results of third-party AI systems used to sell or promote their products and services.
Open-source software and libraries also pose risks that financial institutions can address within established risk management frameworks and controls. These should be subjected to the same governance standards as in-house or vendor models.
AI models increase the dependency on cloud computing, as most AI models are hosted in the cloud or in a hybrid environment. A prolonged disruption could have severe implications, even with protections outlined in service level agreements. Alongside complex data infrastructures and legacy systems, data security in the cloud is another important aspect of AI data governance that demands greater attention.
3.5 Operational and cybersecurity risks
Although AI can help financial institutions realize operational efficiencies, these benefits do not offset risks. As they integrate AI into their processes, procedures, and controls, operational risk also increases. The interconnectedness of systems and data creates complexities that can lead to unforeseen issues and can threaten operational resiliency. Without proper security measures in place, the use of AI could increase the risk of cyber attacks. AI malfunctions can quickly translate into financial risks. Financial institutions must apply sufficiently robust safeguards around their AI systems to ensure resiliency.
Cyber risks can stem from using AI tools internally, by making financial institutions more vulnerable to data poisoning or disclosure of protected information. The UK's Department for Science, Innovation and Technology outlined the practical implications of AI cyber vulnerabilities in its May 2024 report: Cyber Security Risks to Artificial Intelligence. Financial institutions utilizing LLMs that are customized using internal data have heightened risks for unintentional release of consumer data or trade secrets.
Cyber risks are also elevated through complex relationships with third parties. Transmitting data with third-party providers exposes risks of interception attacks, even if the third party does not store data. The opaque nature of these models increases the potential for new, hard-to-anticipate exploits. AI models can be subject to attack vectors such as data poisoning, data leakage, evasion attacks, and model extraction.
4 External risks from AI
There are also emerging systemic risks associated with AI, in that the continual advancement of AI-related technologies could have systemic implications. The proliferation of AI in the broader economy and in the hands of threat actors can introduce new risks to financial stability.
4.1 Reports on rates of technological progress in AI
AI is already performing at human level in many tasks and will keep improving.Footnote 11 Hardware providers are also making significant advances in graphics processing units (GPU) that power gen AI training. So-called "scaling laws" imply that increasing computational resources will enable larger and more powerful models. Researchers are also enhancing algorithms and the quality of training data to improve reliability, reduce inaccurate outputs, and increase capabilities. The major tech companies are all investing heavily to create autonomous AI agents.
4.2 Reports on increasing cyber and fraud threats
The IMF estimates that the cost of severe cyber attacks on financial institutions has quadrupled in recent years. AI is quickly becoming a factor in rising cyber risks, as gen AI can make attacks easier to execute.
Gen AI is increasingly being leveraged by threat actors:
- Deepfakes and voice spoofing can be used for identity theft, bypassing security controls, or perpetrating scams. Globally, 91% of financial institutions are rethinking their use of voice-verification due to AI voice-cloning.
- Identity theft and synthetic identity creation can be used to impersonate individuals or open fraudulent accounts based on fake identities.Footnote 12
- The generation of emails/SMS for phishing/smishing scams can enable spear fishing on a mass scale, where hyper-personalized and believable messages are automatically generated.
- The swift programming of new malware or malware variants can allow threat actors to quickly create new code to exploit vulnerabilities and modify existing attacks.
- Vulnerability identification in financial institutions' networks and systems can significantly shorten the window between discovering vulnerabilities and implementing an attack.
- AI models used by financial institutions can be exploited through data poisoning, data leakage during inference, evasion attacks, and model extraction.
Smaller financial institutions could become more vulnerable to external AI-enabled threats. According to the IMF, smaller financial institutions have traditionally been the target of cyber attacks less frequently than larger ones, due to having a smaller digital footprint and representing smaller payoffs relative to the time required to orchestrate an attack. The ability of bad actors to deploy gen AI, however, can potentially enable much greater automation of attack strategies. This could shift the economics such that attacking smaller institutions might become more attractive to threat actors.
4.3 Business and financial risks
Financial institutions that do not adopt AI may face financial and competitive pressure in the future. If AI begins to disrupt the financial industry, firms that lag in adopting AI may find it difficult to respond without having in-house AI expertise and knowledge.
The uncertainty of future business risks from AI is another challenge. While these risks may be limited at present, they could increase in the future if other financial institutions or new entrants are better able to leverage the evolving technology to gain market share. Leading technology companies are investing heavily in autonomous agents that could eventually reduce different revenue streams that financial institutions currently depend on.
4.4 Emerging credit, market, and liquidity risks
Macroeconomic impacts of AI could lead to credit losses. The IMF estimates that 60% of jobs in advanced economies will be affected by AI automation. Should this materialize into job losses to any appreciable extent, the change could increase retail credit risk for financial institutions. Similarly, exposures to corporate entities could result in credit losses in the years ahead if disruption from AI creates winners and losers across a range of industries.
Market and liquidity risks could also be impacted by AI. US Securities and Exchange Commission (SEC) chair Gary Gensler has been vocal about the risk that AI adoption by hedge funds and other firms could lead to herding behaviour. Flash crashes similar to what was experienced in 2010, caused by algorithmic trading, are also possible. As AI trading models become ever more attuned to leading indicators, AI-driven feedback loops could accelerate volatility across asset classes. Funding liquidity could also be impacted by AI in the foreseeable future. Fintech firms in some countries already allow customers to automatically move deposits between financial institutions to maximize interest earned. If AI agents could do this, the traditional stickiness of retail deposits as a funding source for DTIs might be reduced.
5 Potential pitfalls in AI risk management
- Risk management lagging behind the speed of AI adoption
-
Arguably the greatest risk impact of AI is on overall risk governance. The novelty of the technology requires risk management that is agile and vigilant. This is especially salient in business lines that use AI but that were not traditionally subject to model risk governance.
- Absence of AI risk management oversight
-
Risk management gaps could be exposed if a financial institution only addresses AI risks in individual risk frameworks (for example, in model risk or cyber risk frameworks). Moreover, properly setting up organizational, governance, and operational frameworks can yield other benefits, particularly when taking a multidisciplinary approach, with collaboration and communication across the firm.
- AI controls don't consider all risks across the AI model lifecycle
-
Performing an initial risk assessment on an AI model is a common practice. But if an initial risk assessment is focused on only one risk category, it might miss other risks discussed above. Periodic reassessments could help ensure risks are still being mitigated.
- Lacking contingency actions or safeguards when using AI models
-
The explainability challenges of many AI models increases the chances of them not behaving as expected. Controls can include human-in-the-loop, performance monitoring, back-up systems, alerts, Machine Learning Operations, and limitations on PII use.
- Not updating risk frameworks and controls for gen AI risks
-
Using gen AI models can amplify risks such as explainability, bias, and third‑party dependencies. Employees using publicly accessible third-party gen AI tools is another risk. These concerns can warrant specific gen AI controls, such as enhanced monitoring, employee education on appropriate use, and preventing the input of confidential data into prompts.
- Neglecting to provide sufficient AI training to employees
-
Given that AI is such a fast-evolving space, there is often a general lack of knowledge and expertise. This may translate into wrong decisions or increased risks. More training, at all levels of the organization, is generally beneficial to raise awareness of the risks, provide guidance on appropriate use, and ensure users are aware of applicable limitations and controls. This is particularly true for employees who directly use the technology, and for senior decision makers.
- Not taking a strategic approach to AI adoption
-
Many financial institutions indicate falling behind on technology advances could present business or strategic risks. Others avoid AI activities due to uncertainty. Decisions regarding AI adoption should be the outcome of an informed strategic evaluation balancing the benefits and risks considering each financial institution's circumstances.
- Thinking that not using AI means there are no AI risks
-
Financial institutions that do not use AI should still consider updating their risk frameworks, training, or governance processes to account for AI-related risks. This would help mitigate risks including business risk, risks from inappropriate use of AI tools by employees, and external risks from AI.
6 Regulators also need to keep pace
OSFI and FCAC aim to respond dynamically and proactively to an evolving risk environment, as the uncertain impacts of AI represent a challenge for regulators as well. The AI Questionnaire showed a wide range of practices for risk management and governance, which may indicate the need for more dialogue on operationalizing best practices.
Financial institutions are requesting greater clarity and consistency in regulations. Many of them mentioned they are waiting for the final OSFI E-23 –Enterprise-Wide Model Risk Management Guideline, Bill C-27 Artificial Intelligence and Data Act, and other AI guidelines before fully committing to AI-related actions.
A second financial industry forum on AI is being planned. There was strong presence and commitment from financial institutions, academia, and peer regulators during the first FIFAI sessions, culminating in the 2023 publication. The EDGE principles outlined in the FIFAI Responsible AI report were well received but may not have sufficiently addressed difficult practical challenges and trade-offs. OSFI, in partnership with GRI and industry participants, will aim to build upon prior work in future FIFAI workshops, to establish more specific best practices.
7 Conclusion
Canadian financial institutions have been enthusiastic adopters of AI models and systems in recent years. This trend is poised to continue as they evolve their AI strategy to keep pace with the competition. Financial institutions will continue to face challenges going forward, given the cross-cutting nature of AI adoption risks.
- Financial institutions need to not only conduct risk identification and assessment in a rigorous manner, but also to establish multidisciplinary and diverse teams given the increased scope, scale, and complexity of some AI use cases.
- Financial institutions must also be open, honest, and transparent in dealing with their customers when it comes to AI and data.
- AI will be integrated into more business activities at financial institutions, and in society more broadly. Given the dynamism of this space and the interconnectedness with systems and data as well as with other risks, financial institutions should plan and define an AI strategy even if they do not plan to adopt AI in the short term.
- As a transverse risk, AI adoption needs to be addressed comprehensively, with risk management standards that integrate all related risks. The rapid advancement of AI demands financial institutions be proactive in their risk governance, strategic approaches, and risk management. Boards and oversight bodies must be engaged, to ensure their institutions are properly prepared for AI outcomes, by balancing the benefits and the risks.